Introduction
The CDSE
package for R was developed to allow access to
the ‘Copernicus Data Space
Ecosystem’ data and services from R. The
'Copernicus Data Space Ecosystem'
, deployed in 2023, offers
access to the EO data collection from the Copernicus missions, with
discovery and download capabilities and numerous data processing tools.
In particular, the ‘Sentinel
Hub’ API provides access to the multi-spectral and multi-temporal
big data satellite imagery service, capable of fully automated,
real-time processing and distribution of remote sensing data and related
EO products. Users can use APIs to retrieve satellite data over their
AOI and specific time range from full archives in a matter of seconds.
When working on the application of EO where the area of interest is
relatively small compared to the image tiles distributed by Copernicus
(100 x 100 km), it allows to retrieve just the portion of the image of
interest rather than downloading the huge tile image file and processing
it locally. The goal of the CDSE
package is to provide easy
access to this functionality from R.
The main functions allow to search the catalog of available imagery from the Sentinel-1, Sentinel-2, Sentinel-3, and Sentinel-5 missions, and to process and download the images of an area of interest and a time range in various formats. Other functions might be added in subsequent releases of the package.
API authentication
Most of the API functions require OAuth2 authentication. The
recommended procedure is to obtain an authentication client object from
the GetOAuthClient
function and pass it as the
client
argument to the functions requiring the
authentication. For more detailed information, you are invited to
consult the “Before you start
” document.
id <- Sys.getenv("CDSE_ID")
secret <- Sys.getenv("CDSE_SECRET")
OAuthClient <- GetOAuthClient(id = id, secret = secret)
Collections
We can get the list of all the imagery collections available in the
'Copernicus Data Space Ecosystem'
. By default, the list is
formatted as a data.frame listing the main collection features. It is
also possible to obtain the raw list with all information by setting the
argument as_data_frame
to FALSE
.
collections <- GetCollections(as_data_frame = TRUE)
collections
#> id title
#> 1 sentinel-2-l1c Sentinel 2 L1C
#> 2 sentinel-3-olci-l2 Sentinel 3 OLCI L2
#> 3 sentinel-3-olci Sentinel 3 OLCI
#> 4 sentinel-3-slstr Sentinel 3 SLSTR
#> 5 sentinel-1-grd Sentinel 1 GRD
#> 6 sentinel-2-l2a Sentinel 2 L2A
#> 7 sentinel-5p-l2 Sentinel 5 Precursor
#> description
#> 1 Sentinel 2 imagery processed to level 1C
#> 2 Sentinel 3 data derived from imagery captured by OLCI sensor
#> 3 Sentinel 3 imagery captured by OLCI sensor
#> 4 Sentinel 3 imagery captured by SLSTR sensor
#> 5 Sentinel 1 Ground Range Detected Imagery
#> 6 Sentinel 2 imagery processed to level 2A
#> 7 Sentinel 5 Precursor imagery captured by TROPOMI sensor
#> since instrument gsd bands constellation long.min lat.min
#> 1 2015-11-01T00:00:00Z msi 10 13 sentinel-2 -180 -56
#> 2 2016-04-17T11:33:13Z olci 300 NA <NA> -180 -85
#> 3 2016-04-17T11:33:13Z olci 300 21 <NA> -180 -85
#> 4 2016-04-17T11:33:13Z slstr 1000 11 <NA> -180 -85
#> 5 2014-10-03T00:00:00Z c-sar NA NA sentinel-1 -180 -85
#> 6 2016-11-01T00:00:00Z msi 10 12 sentinel-2 -180 -56
#> 7 2018-04-30T00:18:50Z tropomi 5500 NA <NA> -180 -85
#> long.max lat.max
#> 1 180 83
#> 2 180 85
#> 3 180 85
#> 4 180 85
#> 5 180 85
#> 6 180 83
#> 7 180 85
Catalog search
The imagery catalog can be searched by spatial and temporal extent
for every collection present in the
'Copernicus Data Space Ecosystem'
. For the spatial filter,
you can provide either a sf
or sfc
object from
the sf
package, typically a (multi)polygon, describing the
Area of Interest, or a numeric vector of four elements describing the
bounding box of interest. For the temporal filter, you must specify the
time range by either Date
or character
values
that can be converted to date by as.Date
function. Open
intervals (one side only) can be obtained by providing the
NA
or NULL
value for the corresponding
argument.
dsn <- system.file("extdata", "luxembourg.geojson", package = "CDSE")
aoi <- sf::read_sf(dsn, as_tibble = FALSE)
images <- SearchCatalog(aoi = aoi, from = "2023-07-01", to = "2023-07-31",
collection = "sentinel-2-l2a", with_geometry = TRUE, client = OAuthClient)
images
#> Simple feature collection with 70 features and 11 fields
#> Geometry type: POLYGON
#> Dimension: XY
#> Bounding box: xmin: 4.357925 ymin: 48.58836 xmax: 7.775117 ymax: 50.54532
#> Geodetic CRS: WGS 84
#> First 10 features:
#> acquisitionDate tileCloudCover areaCoverage satellite
#> 1 2023-07-31 98.87 1.845 sentinel-2a
#> 2 2023-07-31 99.85 20.346 sentinel-2a
#> 3 2023-07-31 99.74 5.934 sentinel-2a
#> 4 2023-07-31 99.94 16.324 sentinel-2a
#> 5 2023-07-31 99.91 92.463 sentinel-2a
#> 6 2023-07-31 99.41 22.236 sentinel-2a
#> 7 2023-07-28 99.99 4.986 sentinel-2a
#> 8 2023-07-28 99.99 5.659 sentinel-2a
#> 9 2023-07-28 99.99 4.294 sentinel-2a
#> 10 2023-07-28 100.00 6.855 sentinel-2a
#> acquisitionTimestampUTC acquisitionTimestampLocal
#> 1 2023-07-31 10:47:29 2023-07-31 12:47:29
#> 2 2023-07-31 10:47:25 2023-07-31 12:47:25
#> 3 2023-07-31 10:47:23 2023-07-31 12:47:23
#> 4 2023-07-31 10:47:14 2023-07-31 12:47:14
#> 5 2023-07-31 10:47:11 2023-07-31 12:47:11
#> 6 2023-07-31 10:47:09 2023-07-31 12:47:09
#> 7 2023-07-28 10:37:28 2023-07-28 12:37:28
#> 8 2023-07-28 10:37:27 2023-07-28 12:37:27
#> 9 2023-07-28 10:37:21 2023-07-28 12:37:21
#> 10 2023-07-28 10:37:20 2023-07-28 12:37:20
#> sourceId long.min
#> 1 S2A_MSIL2A_20230731T103631_N0509_R008_T31UFQ_20230731T202459.SAFE 4.357925
#> 2 S2A_MSIL2A_20230731T103631_N0509_R008_T31UGQ_20230731T202459.SAFE 5.714101
#> 3 S2A_MSIL2A_20230731T103631_N0509_R008_T32ULV_20230731T202459.SAFE 6.230946
#> 4 S2A_MSIL2A_20230731T103631_N0509_R008_T31UFR_20230731T202459.SAFE 4.382698
#> 5 S2A_MSIL2A_20230731T103631_N0509_R008_T31UGR_20230731T202459.SAFE 5.763559
#> 6 S2A_MSIL2A_20230731T103631_N0509_R008_T32ULA_20230731T202459.SAFE 6.178682
#> 7 S2A_MSIL2A_20230728T102601_N0509_R108_T31UGQ_20230728T180355.SAFE 5.880056
#> 8 S2A_MSIL2A_20230728T102601_N0509_R108_T32ULV_20230728T180355.SAFE 6.234494
#> 9 S2A_MSIL2A_20230728T102601_N0509_R108_T31UGQ_20230728T164605.SAFE 6.218396
#> 10 S2A_MSIL2A_20230728T102601_N0509_R108_T31UGR_20230728T180355.SAFE 6.219516
#> lat.min long.max lat.max geometry
#> 1 48.62983 5.904558 49.64443 POLYGON ((4.385187 49.64443...
#> 2 48.58836 7.285031 49.61960 POLYGON ((5.768528 49.6196,...
#> 3 48.63394 7.762836 49.64597 POLYGON ((6.230946 49.61959...
#> 4 49.52843 5.959371 50.54373 POLYGON ((4.411363 50.54373...
#> 5 49.48563 7.365728 50.51810 POLYGON ((5.820783 50.5181,...
#> 6 49.53174 7.752769 50.54532 POLYGON ((6.178682 50.51809...
#> 7 48.58836 7.281682 49.60696 POLYGON ((6.254039 49.60696...
#> 8 48.63304 7.775117 49.62896 POLYGON ((6.259211 49.62026...
#> 9 49.36204 7.285031 49.60696 POLYGON ((6.254038 49.60696...
#> 10 49.48568 7.279271 49.67956 POLYGON ((7.27598 49.48568,...
We can visualize the coverage of the area of interest by the satellite image tiles by plotting the footprints of the available images and showing the region of interest in red.
library(maps)
days <- range(as.Date(images$acquisitionDate))
maps::map(database = "world", col = "lightgrey", fill = TRUE, mar = c(0, 0, 4, 0),
xlim = c(3, 9), ylim = c(47.5, 51.5))
plot(sf::st_geometry(aoi), add = TRUE, col = "red", border = FALSE)
plot(sf::st_geometry(images), add = TRUE)
title(main = sprintf("AOI coverage by image tiles for period %s",
paste(days, collapse = " / ")), line = 1L, cex.main = 0.75)
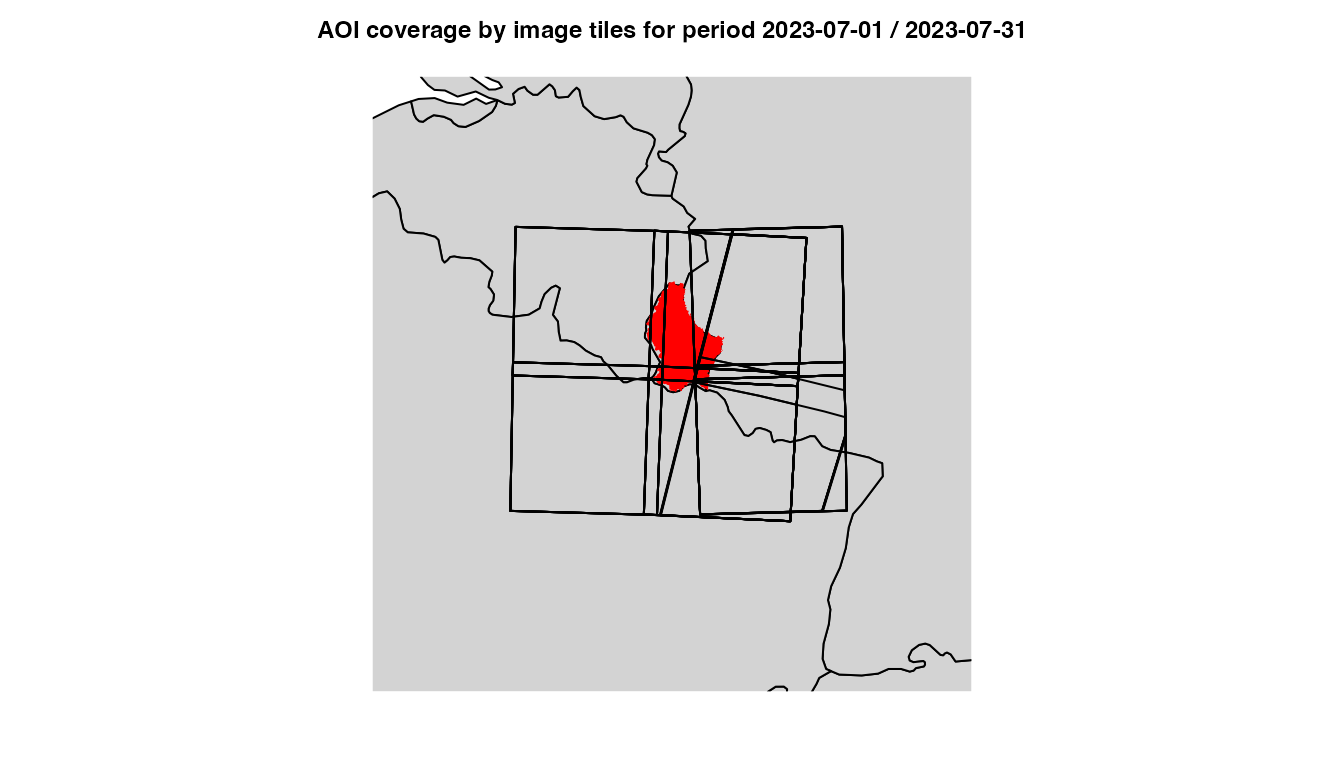
Luxembourg image tiles coverage
Some tiles cover only a small fraction of the area of interest, while others cover almost the entire area.
summary(images$areaCoverage)
#> Min. 1st Qu. Median Mean 3rd Qu. Max.
#> 1.845 5.603 15.113 19.758 20.346 92.463
The tile number can be obtained from the image attribute
sourceId
, as explained here. We can
therefore summarize the distribution of area coverage by tile number,
and see which tiles provide the best coverage of the AOI.
tileNumber <- substring(images$sourceId, 39, 44)
by(images$areaCoverage, INDICES = tileNumber, FUN = summary)
#> tileNumber: T31UFQ
#> Min. 1st Qu. Median Mean 3rd Qu. Max.
#> 1.845 1.845 1.845 1.845 1.845 1.845
#> ------------------------------------------------------------
#> tileNumber: T31UFR
#> Min. 1st Qu. Median Mean 3rd Qu. Max.
#> 16.32 16.32 16.32 16.32 16.32 16.32
#> ------------------------------------------------------------
#> tileNumber: T31UGQ
#> Min. 1st Qu. Median Mean 3rd Qu. Max.
#> 4.294 4.909 12.706 12.586 20.346 20.346
#> ------------------------------------------------------------
#> tileNumber: T31UGR
#> Min. 1st Qu. Median Mean 3rd Qu. Max.
#> 6.855 15.607 54.299 53.426 92.463 92.463
#> ------------------------------------------------------------
#> tileNumber: T32ULA
#> Min. 1st Qu. Median Mean 3rd Qu. Max.
#> 6.169 14.950 18.814 17.972 22.236 22.236
#> ------------------------------------------------------------
#> tileNumber: T32ULV
#> Min. 1st Qu. Median Mean 3rd Qu. Max.
#> 4.944 5.603 5.819 5.723 5.934 5.934
Catalog by season
Sometimes one can be interested in only a given period of each year,
for example, the images taken during the summer months (June to August).
We can filter an existing image catalog a posteriori using the
SeasonalFilter
function.
dsn <- system.file("extdata", "centralpark.geojson", package = "CDSE")
aoi <- sf::read_sf(dsn, as_tibble = FALSE)
images <- SearchCatalog(aoi = aoi, from = "2021-01-01", to = "2023-12-31",
collection = "sentinel-2-l2a", with_geometry = FALSE, filter = "eo:cloud_cover < 5",
client = OAuthClient)
dim(images)
#> [1] 77 10
summer_images <- SeasonalFilter(images, from = "2021-06-01", to = "2023-08-31")
dim(summer_images)
#> [1] 14 10
It is also possible to query the API directly on the desired seasonal
periods by using a vectorized version of the SearchCatalog
function. The vectorized versions allow running a series of queries
having the same parameter values except for either time range, AOI, or
the bounding box parameters, using lapply
or similar
function, and thus potentially also using the parallel processing.
dsn <- system.file("extdata", "centralpark.geojson", package = "CDSE")
aoi <- sf::read_sf(dsn, as_tibble = FALSE)
seasons <- SeasonalTimerange(from = "2021-06-01", to = "2023-08-31")
lst_summer_images <- lapply(seasons, SearchCatalogByTimerange, aoi = aoi,
collection = "sentinel-2-l2a", filter = "eo:cloud_cover < 5", with_geometry = FALSE,
client = OAuthClient)
summer_images <- do.call(rbind, lst_summer_images)
dim(summer_images)
#> [1] 14 10
summer_images <- summer_images[rev(order(summer_images$acquisitionDate)), ]
row.names(summer_images) <- NULL
summer_images
#> acquisitionDate tileCloudCover satellite acquisitionTimestampUTC
#> 1 2023-08-20 0.03 sentinel-2a 2023-08-20 15:51:57
#> 2 2023-07-31 3.96 sentinel-2a 2023-07-31 15:51:56
#> 3 2023-07-26 0.89 sentinel-2b 2023-07-26 15:51:56
#> 4 2023-07-11 4.49 sentinel-2a 2023-07-11 15:51:56
#> 5 2023-06-01 0.00 sentinel-2a 2023-06-01 15:51:54
#> 6 2022-08-25 1.69 sentinel-2a 2022-08-25 15:52:03
#> 7 2022-08-03 4.58 sentinel-2b 2022-08-03 16:01:51
#> 8 2022-07-19 1.37 sentinel-2a 2022-07-19 16:01:58
#> 9 2022-07-11 4.89 sentinel-2b 2022-07-11 15:51:57
#> 10 2022-06-19 1.91 sentinel-2a 2022-06-19 16:01:58
#> 11 2022-06-06 1.00 sentinel-2a 2022-06-06 15:51:58
#> 12 2022-06-04 2.76 sentinel-2b 2022-06-04 16:01:47
#> 13 2021-06-16 4.74 sentinel-2b 2021-06-16 15:51:51
#> 14 2021-06-06 0.38 sentinel-2b 2021-06-06 15:51:52
#> acquisitionTimestampLocal
#> 1 2023-08-20 11:51:57
#> 2 2023-07-31 11:51:56
#> 3 2023-07-26 11:51:56
#> 4 2023-07-11 11:51:56
#> 5 2023-06-01 11:51:54
#> 6 2022-08-25 11:52:03
#> 7 2022-08-03 12:01:51
#> 8 2022-07-19 12:01:58
#> 9 2022-07-11 11:51:57
#> 10 2022-06-19 12:01:58
#> 11 2022-06-06 11:51:58
#> 12 2022-06-04 12:01:47
#> 13 2021-06-16 11:51:51
#> 14 2021-06-06 11:51:52
#> sourceId long.min
#> 1 S2A_MSIL2A_20230820T153821_N0509_R011_T18TWL_20230820T221958.SAFE -74.89340
#> 2 S2A_MSIL2A_20230731T155141_N0509_R011_T18TWL_20230731T220001.SAFE -74.88632
#> 3 S2B_MSIL2A_20230726T153819_N0509_R011_T18TWL_20230726T175732.SAFE -74.88431
#> 4 S2A_MSIL2A_20230711T153821_N0509_R011_T18TWL_20230711T233752.SAFE -74.88773
#> 5 S2A_MSIL2A_20230601T155141_N0509_R011_T18TWL_20230601T220554.SAFE -74.89199
#> 6 S2A_MSIL2A_20220825T155151_N0400_R011_T18TWL_20220825T220800.SAFE -74.89577
#> 7 S2B_MSIL2A_20220803T154819_N0400_R054_T18TWL_20220804T065759.SAFE -75.00023
#> 8 S2A_MSIL2A_20220719T154951_N0400_R054_T18TWL_20220719T234155.SAFE -75.00023
#> 9 S2B_MSIL2A_20220711T153819_N0400_R011_T18TWL_20220711T182252.SAFE -74.88525
#> 10 S2A_MSIL2A_20220619T154821_N0400_R054_T18TWL_20220619T232314.SAFE -75.00023
#> 11 S2A_MSIL2A_20220606T153821_N0400_R011_T18TWL_20220606T230911.SAFE -74.88136
#> 12 S2B_MSIL2A_20220604T154809_N0400_R054_T18TWL_20220604T201432.SAFE -75.00023
#> 13 S2B_MSIL2A_20210616T153809_N0500_R011_T18TWL_20230131T171455.SAFE -74.88136
#> 14 S2B_MSIL2A_20210606T153809_N0500_R011_T18TWL_20230603T225907.SAFE -74.88277
#> lat.min long.max lat.max
#> 1 40.55548 -73.68381 41.55110
#> 2 40.55548 -73.68381 41.55107
#> 3 40.55548 -73.68381 41.55107
#> 4 40.55548 -73.68381 41.55108
#> 5 40.55548 -73.68381 41.55109
#> 6 40.55548 -73.68381 41.55111
#> 7 40.55820 -73.68381 41.55184
#> 8 40.55819 -73.68381 41.55184
#> 9 40.55550 -73.69200 41.35177
#> 10 40.55681 -73.68381 41.55184
#> 11 40.55548 -73.68381 41.55105
#> 12 40.55781 -73.68381 41.55184
#> 13 40.55548 -73.68381 41.55106
#> 14 40.55548 -73.68381 41.55106
Scripts
As we shall see in the examples below, we have to provide a
script
argument to the GetArchiveImage
function.
An evalscript (or “custom script”) is a piece of JavaScript code that defines how the satellite data shall be processed by the API and what values the service shall return. It is a required part of any request involving data processing, such as retrieving an image of the area of interest.
The evaluation scripts can use any JavaScript function or language structures, along with certain utility functions provided by the API for user convenience. Chrome V8 JavaScript engine is used for running the evalscripts.
The evaluation scripts are passed as the script
argument
to the GetArchiveImage
function. It has to be either a
character string containing the evaluation script or the name of the
file containing the script. The scripts
folder of this
package contains a few examples of evaluation scripts.
It is beyond the scope of this document to provide guidance for writing scripts, we encourage users to consult the API Beginners Guide and Evalscript (custom script) documentation. You can find a big collection of custom scripts that you can readily use in this repository.
Retrieving images
Retrieving AOI satellite image as a raster object
One of the most important features of the API is its ability to extract only the part of the images covering the area of interest. If the AOI is small as in the example below, this is a significant gain in efficiency (download, local processing) compared to getting the whole tile image and processing it locally.
dsn <- system.file("extdata", "centralpark.geojson", package = "CDSE")
aoi <- sf::read_sf(dsn, as_tibble = FALSE)
images <- SearchCatalog(aoi = aoi, from = "2021-05-01", to = "2021-05-31",
collection = "sentinel-2-l2a", with_geometry = TRUE, client = OAuthClient)
images
#> Simple feature collection with 12 features and 11 fields
#> Geometry type: POLYGON
#> Dimension: XY
#> Bounding box: xmin: -75.00023 ymin: 40.55548 xmax: -73.68381 ymax: 41.55184
#> Geodetic CRS: WGS 84
#> First 10 features:
#> acquisitionDate tileCloudCover areaCoverage satellite
#> 1 2021-05-30 100.00 100 sentinel-2b
#> 2 2021-05-27 16.26 100 sentinel-2b
#> 3 2021-05-25 26.47 100 sentinel-2a
#> 4 2021-05-22 100.00 100 sentinel-2a
#> 5 2021-05-20 24.31 100 sentinel-2b
#> 6 2021-05-17 7.17 100 sentinel-2b
#> 7 2021-05-15 28.17 100 sentinel-2a
#> 8 2021-05-12 1.35 100 sentinel-2a
#> 9 2021-05-10 92.67 100 sentinel-2b
#> 10 2021-05-07 89.62 100 sentinel-2b
#> acquisitionTimestampUTC acquisitionTimestampLocal
#> 1 2021-05-30 16:01:47 2021-05-30 12:01:47
#> 2 2021-05-27 15:51:51 2021-05-27 11:51:51
#> 3 2021-05-25 16:01:47 2021-05-25 12:01:47
#> 4 2021-05-22 15:51:51 2021-05-22 11:51:51
#> 5 2021-05-20 16:01:47 2021-05-20 12:01:47
#> 6 2021-05-17 15:51:50 2021-05-17 11:51:50
#> 7 2021-05-15 16:01:47 2021-05-15 12:01:47
#> 8 2021-05-12 15:51:50 2021-05-12 11:51:50
#> 9 2021-05-10 16:01:45 2021-05-10 12:01:45
#> 10 2021-05-07 15:51:48 2021-05-07 11:51:48
#> sourceId long.min
#> 1 S2B_MSIL2A_20210530T154809_N0500_R054_T18TWL_20230219T165456.SAFE -75.00023
#> 2 S2B_MSIL2A_20210527T153809_N0500_R011_T18TWL_20230603T173050.SAFE -74.88348
#> 3 S2A_MSIL2A_20210525T154911_N0500_R054_T18TWL_20230208T124728.SAFE -75.00023
#> 4 S2A_MSIL2A_20210522T153911_N0500_R011_T18TWL_20230604T065046.SAFE -74.87781
#> 5 S2B_MSIL2A_20210520T154809_N0500_R054_T18TWL_20230219T225246.SAFE -75.00023
#> 6 S2B_MSIL2A_20210517T153809_N0500_R011_T18TWL_20230603T145938.SAFE -74.88206
#> 7 S2A_MSIL2A_20210515T154911_N0500_R054_T18TWL_20230206T101926.SAFE -75.00023
#> 8 S2A_MSIL2A_20210512T153911_N0500_R011_T18TWL_20230604T080336.SAFE -74.87710
#> 9 S2B_MSIL2A_20210510T154809_N0500_R054_T18TWL_20230208T070834.SAFE -75.00023
#> 10 S2B_MSIL2A_20210507T153809_N0500_R011_T18TWL_20230604T114048.SAFE -74.87639
#> lat.min long.max lat.max geometry
#> 1 40.55822 -73.68381 41.55184 POLYGON ((-75.00023 41.5518...
#> 2 40.55548 -73.68381 41.55106 POLYGON ((-74.57884 41.5510...
#> 3 40.55811 -73.68381 41.55184 POLYGON ((-75.00023 41.5518...
#> 4 40.55548 -73.68381 41.55104 POLYGON ((-74.57309 41.5510...
#> 5 40.55775 -73.68381 41.55184 POLYGON ((-75.00023 41.5518...
#> 6 40.55548 -73.68381 41.55106 POLYGON ((-74.57668 41.5510...
#> 7 40.55775 -73.68381 41.55184 POLYGON ((-75.00023 41.5518...
#> 8 40.55548 -73.68381 41.55104 POLYGON ((-74.57165 41.5510...
#> 9 40.55815 -73.68381 41.55184 POLYGON ((-75.00023 41.5518...
#> 10 40.55548 -73.68381 41.55103 POLYGON ((-74.57021 41.5510...
summary(images$areaCoverage)
#> Min. 1st Qu. Median Mean 3rd Qu. Max.
#> 100 100 100 100 100 100
As the area is small, it is systematically fully covered by all
available images. We shall select the date with the least cloud cover,
and retrieve the NDVI values as a SpatRaster
from package
terra
. This allows further processing of the data, as shown
below by replacing all negative values with zero. The size of the pixels
is specified directly by the resolution
argument. We are
also adding a 100-meter buffer
around the area of interest
and mask
ing the pixels outside of the AOI.
day <- images[order(images$tileCloudCover), ]$acquisitionDate[1]
script_file <- system.file("scripts", "NDVI_float32.js", package = "CDSE")
ras <- GetImage(aoi = aoi, time_range = day, script = script_file,
collection = "sentinel-2-l2a", format = "image/tiff", mosaicking_order = "leastCC",
resolution = 10, mask = TRUE, buffer = 100, client = OAuthClient)
ras
#> class : SpatRaster
#> dimensions : 383, 355, 1 (nrow, ncol, nlyr)
#> resolution : 0.0001003292, 0.0001003292 (x, y)
#> extent : -73.98355, -73.94794, 40.76322, 40.80165 (xmin, xmax, ymin, ymax)
#> coord. ref. : lon/lat WGS 84 (EPSG:4326)
#> source(s) : memory
#> name : file31eb642c7e71
#> min value : -0.5069648
#> max value : 0.9507549
ras[ras < 0] <- 0
terra::plot(ras, main = paste("Central Park NDVI on", day), cex.main = 0.75,
col = colorRampPalette(c("darkred", "yellow", "darkgreen"))(99))
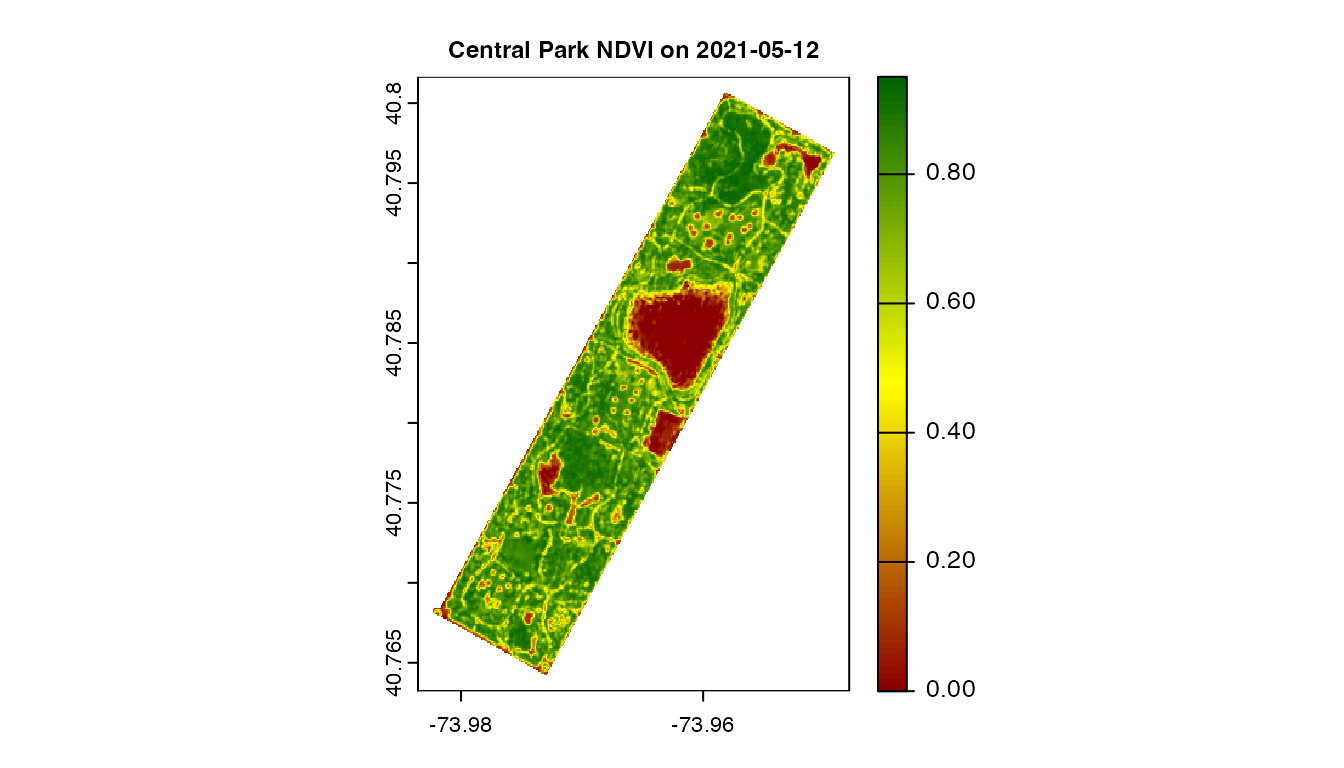
Central Park NDVI raster
Retrieving AOI satellite image as an image file
If we don’t want to process the satellite image locally but simply use it as an image file (to include in a report or a Web page, for example), we can use the appropriate script that will render a three-band raster for RGB layers (or one for black-and-white image). Here we specify the area of interest by its bounding box instead of the exact geometry. We also demonstrate that the evaluation script can be passed as a single character string, and provide the number of pixels in the output image rather than the size of individual pixels - it makes more sense if the image is intended for display and not processing.
bbox <- as.numeric(sf::st_bbox(aoi))
script_text <- paste(readLines(system.file("scripts", "TrueColorS2L2A.js",
package = "CDSE")), collapse = "\n")
cat(c(readLines(system.file("scripts", "TrueColorS2L2A.js", package = "CDSE"), n = 15),
"..."), sep = "\n")
#> //VERSION=3
#> //Optimized Sentinel-2 L2A True Color
#>
#> function setup() {
#> return {
#> input: ["B04", "B03", "B02", "dataMask"],
#> output: { bands: 4 }
#> };
#> }
#>
#> function evaluatePixel(smp) {
#> const rgbLin = satEnh(sAdj(smp.B04), sAdj(smp.B03), sAdj(smp.B02));
#> return [sRGB(rgbLin[0]), sRGB(rgbLin[1]), sRGB(rgbLin[2]), smp.dataMask];
#> }
#>
#> ...
png <- tempfile("img", fileext = ".png")
GetImage(bbox = bbox, time_range = day, script = script_text,
collection = "sentinel-2-l2a", file = png, format = "image/png",
mosaicking_order = "leastCC", pixels = c(600, 950), client = OAuthClient)
terra::plotRGB(terra::rast(png))
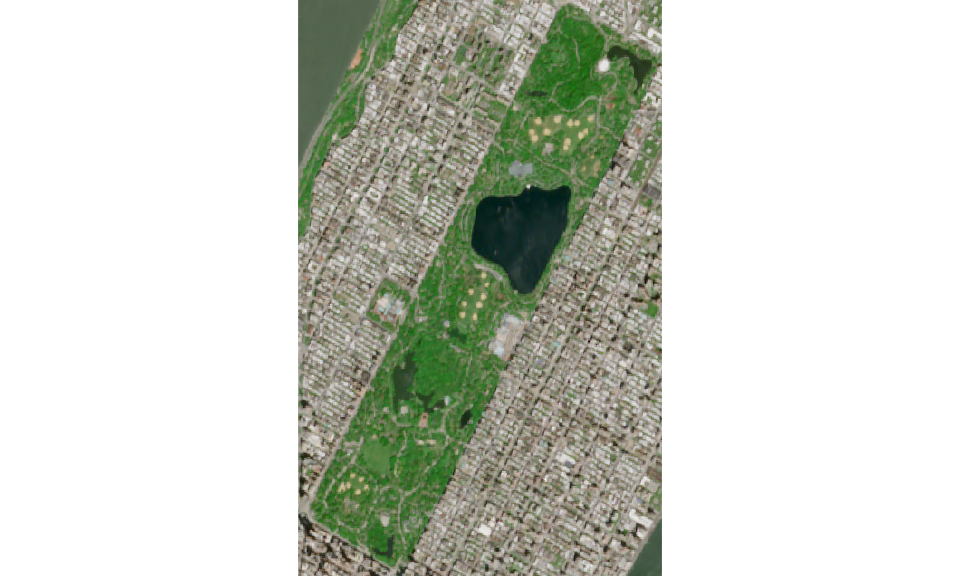
Central Park image as PNG file
Retrieving a series of images in a batch
It often happens that one is interested in acquiring a series of
images of a particular zone (AOI or bounding box) for several dates, or
the images of different areas of interest for the same date (probably
located close to each other so that they are visited on the same day).
The GetImageBy*
functions
(GetImageByTimerange
, GetImageByAOI
,
GetImageByBbox
) facilitate this task as they are
specifically crafted for being called from a lapply
-like
function, and thus potentially be executed in parallel. We shall
illustrate how to do this with an example.
dsn <- system.file("extdata", "centralpark.geojson", package = "CDSE")
aoi <- sf::read_sf(dsn, as_tibble = FALSE)
images <- SearchCatalog(aoi = aoi, from = "2023-01-01", to = "2023-12-31",
collection = "sentinel-2-l2a", with_geometry = TRUE,
filter = "eo:cloud_cover < 5", client = OAuthClient)
# Get the day with the minimal cloud cover for every month -----------------------------
tmp1 <- images[, c("tileCloudCover", "acquisitionDate")]
tmp1$month <- lubridate::month(images$acquisitionDate)
agg1 <- stats::aggregate(tileCloudCover ~ month, data = tmp1, FUN = min)
tmp2 <- merge.data.frame(agg1, tmp1, by = c("month", "tileCloudCover"), sort = FALSE)
# in case of ties, get an arbitrary date (here the smallest acquisitionDate,
# could also be the biggest)
agg2 <- stats::aggregate(acquisitionDate ~ month, data = tmp2, FUN = min)
monthly <- merge.data.frame(agg2, tmp2, by = c("acquisitionDate", "month"), sort = FALSE)
days <- monthly$acquisitionDate
# Retrieve images in parallel ----------------------------------------------------------
script_file <- system.file("scripts", "NDVI_float32.js", package = "CDSE")
tmp_folder <- tempfile("dir")
if (!dir.exists(tmp_folder)) dir.create(tmp_folder)
cl <- parallel::makeCluster(4)
ans <- parallel::clusterExport(cl, list("tmp_folder"), envir = environment())
ans <- parallel::clusterEvalQ(cl, {library(CDSE)})
lstRast <- parallel::parLapply(cl, days, fun = function(x, ...) {
GetImageByTimerange(x, file = sprintf("%s/img_%s.tiff", tmp_folder, x), ...)},
aoi = aoi, collection = "sentinel-2-l2a", script = script_file,
format = "image/tiff", mosaicking_order = "mostRecent", resolution = 10,
buffer = 0, mask = TRUE, client = OAuthClient)
parallel::stopCluster(cl)
# Plot the images ----------------------------------------------------------------------
par(mfrow = c(3, 4))
ans <- sapply(seq_along(days), FUN = function(i) {
ras <- terra::rast(lstRast[[i]])
day <- days[i]
ras[ras < 0] <- 0
terra::plot(ras, main = paste("Central Park NDVI on", day), range = c(0, 1),
cex.main = 0.7, pax = list(cex.axis = 0.5), plg = list(cex = 0.5),
col = colorRampPalette(c("darkred", "yellow", "darkgreen"))(99))
})
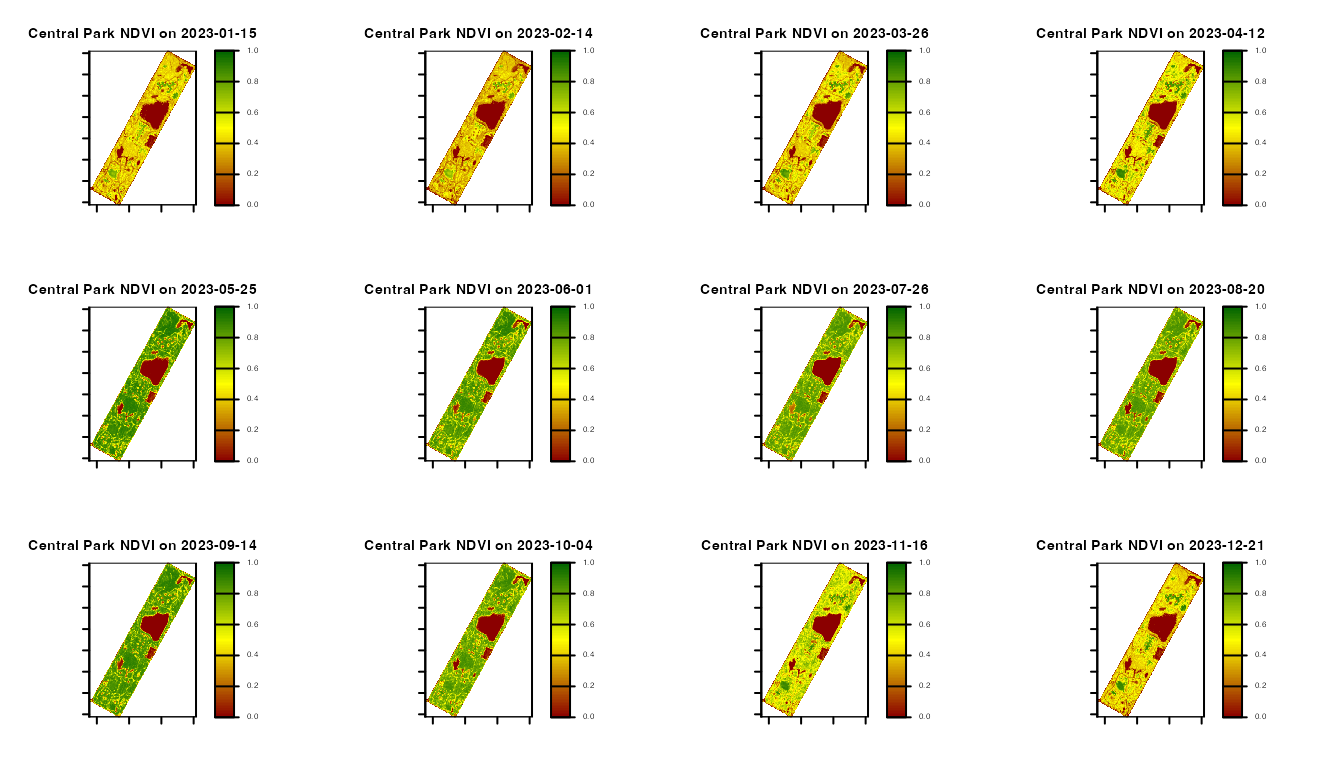
Central Park monthly NDVI
In this particular example, parallelization is not necessarily
beneficial as we are retrieving only 12 images, but for a large number
of images, it can significantly reduce the execution time. Also note
that we have used the filter
argument of the
SearchCatalog
function to limit the list of images to the
tiles having cloud cover < 5%.
Retrieving statistics
If you are only interested in calculating the average value (or some
other statistic) of some index or just the raw band values, the Statistical
API enables you to get statistics calculated based on satellite
imagery without having to download images. You need to specify your area
of interest, time period, evalscript, and which statistical measures
should be calculated. The requested statistics are returned as a
data.frame
or as a list
.
Statistical evalscripts
All general rules for building evalscripts apply. However, there are some specifics when using evalscripts with the Statistical API:
The
evaluatePixel()
function must, in addition to other output, always return alsodataMask
output. This output defines which pixels are excluded from calculations. For more details and an example, see here.The default value of
sampleType
isFLOAT32
.The output.bands parameter in the
setup()
function can be an array. This makes it possible to specify custom names for the output bands and different outputdataMask
for different outputs.
Retrieving simple statistics
Besides the time range, you have to specify the way you want the
values to be aggregated over time. For this you use the
aggregation_period
and aggregation_unit
arguments. The aggregation_unit
must be one of
day
, week
, month
or
year
, and the aggregation_period
providing the
number of aggregation_units
(days, weeks, …) over which the
statistics are calculated. The default values are “1” and “day”,
producing the daily statistics. If the last interval in the given time
range isn’t divisible by the provided aggregation interval, you can skip
the last interval (default behavior), shorten the last interval so that
it ends at the end of the provided time range, or extend the last
interval over the end of the provided time range so that all intervals
are of equal duration. This is controlled by the value of the
lastIntervalBehavior
argument.
dsn <- system.file("extdata", "centralpark.geojson", package = "CDSE")
aoi <- sf::read_sf(dsn, as_tibble = FALSE)
script_file <- system.file("scripts", "NDVI_CLOUDS_STAT.js", package = "CDSE")
daily_stats <- GetStatistics(aoi = aoi, time_range = c("2023-07-01", "2023-07-31"),
collection = "sentinel-2-l2a", script = script_file, mosaicking_order = "leastCC",
resolution = 100, aggregation_period = 1, client = OAuthClient)
weekly_stats <- GetStatistics(aoi = aoi, time_range = c("2023-07-01", "2023-07-31"),
collection = "sentinel-2-l2a", script = script_file,mosaicking_order = "leastCC",
resolution = 100, aggregation_period = 7, client = OAuthClient)
weekly_stats_extended <- GetStatistics(aoi = aoi,
time_range = c("2023-07-01", "2023-07-31"), collection = "sentinel-2-l2a",
script = script_file, mosaicking_order = "leastCC", resolution = 100,
aggregation_period = 1, aggregation_unit = "w", lastIntervalBehavior = "EXTEND",
client = OAuthClient)
daily_stats
#> date output band min mean max stDev
#> 1 2023-07-01 statistics ndvi_value 0 0.210216716 6.509659e-01 1.700419e-01
#> 2 2023-07-01 statistics cloud_cover 0 21.028846154 5.900000e+01 1.413258e+01
#> 3 2023-07-04 statistics ndvi_value 0 0.001150263 7.140577e-02 6.540354e-03
#> 4 2023-07-04 statistics cloud_cover 65 65.000000000 6.500000e+01 0.000000e+00
#> 5 2023-07-06 statistics ndvi_value 0 0.590745587 9.372641e-01 3.130056e-01
#> 6 2023-07-06 statistics cloud_cover 0 0.040865385 1.000000e+00 1.979783e-01
#> 7 2023-07-09 statistics ndvi_value 0 0.000207250 2.914758e-03 5.075671e-04
#> 8 2023-07-09 statistics cloud_cover 65 65.000000000 6.500000e+01 0.000000e+00
#> 9 2023-07-11 statistics ndvi_value 0 0.581748619 9.156258e-01 3.050726e-01
#> 10 2023-07-11 statistics cloud_cover 0 0.031250000 1.000000e+00 1.739926e-01
#> 11 2023-07-14 statistics ndvi_value 0 0.564096934 9.704262e-01 3.135816e-01
#> 12 2023-07-14 statistics cloud_cover 0 1.117788462 4.400000e+01 4.049344e+00
#> 13 2023-07-16 statistics ndvi_value 0 0.011152588 3.966460e-02 5.850098e-03
#> 14 2023-07-16 statistics cloud_cover 65 73.283653846 1.000000e+02 1.457742e+01
#> 15 2023-07-19 statistics ndvi_value 0 0.000000000 0.000000e+00 0.000000e+00
#> 16 2023-07-19 statistics cloud_cover 65 65.000000000 6.500000e+01 0.000000e+00
#> 17 2023-07-21 statistics ndvi_value 0 0.341615600 8.719050e-01 2.366125e-01
#> 18 2023-07-21 statistics cloud_cover 0 18.954326923 1.000000e+02 2.746379e+01
#> 19 2023-07-24 statistics ndvi_value 0 0.318054891 6.846920e-01 1.865030e-01
#> 20 2023-07-24 statistics cloud_cover 0 7.204326923 6.500000e+01 1.404899e+01
#> 21 2023-07-26 statistics ndvi_value 0 0.549814982 8.600247e-01 2.815753e-01
#> 22 2023-07-26 statistics cloud_cover 0 0.040865385 1.000000e+00 1.979783e-01
#> 23 2023-07-29 statistics ndvi_value 0 0.361753207 8.230490e-01 2.353884e-01
#> 24 2023-07-29 statistics cloud_cover 0 14.906250000 6.500000e+01 2.139831e+01
#> 25 2023-07-31 statistics ndvi_value 0 0.574829539 9.039569e-01 2.998643e-01
#> 26 2023-07-31 statistics cloud_cover 0 0.024038462 1.000000e+00 1.531686e-01
#> sampleCount noDataCount
#> 1 1120 704
#> 2 1120 704
#> 3 1120 704
#> 4 1120 704
#> 5 1120 704
#> 6 1120 704
#> 7 1120 704
#> 8 1120 704
#> 9 1120 704
#> 10 1120 704
#> 11 1120 704
#> 12 1120 704
#> 13 1120 704
#> 14 1120 704
#> 15 1120 704
#> 16 1120 704
#> 17 1120 704
#> 18 1120 704
#> 19 1120 704
#> 20 1120 704
#> 21 1120 704
#> 22 1120 704
#> 23 1120 704
#> 24 1120 704
#> 25 1120 704
#> 26 1120 704
weekly_stats
#> from to output band min mean max
#> 1 2023-07-01 2023-07-07 statistics ndvi_value 0 0.59074559 0.9372641
#> 2 2023-07-01 2023-07-07 statistics cloud_cover 0 0.04086538 1.0000000
#> 3 2023-07-08 2023-07-14 statistics ndvi_value 0 0.58174862 0.9156258
#> 4 2023-07-08 2023-07-14 statistics cloud_cover 0 0.03125000 1.0000000
#> 5 2023-07-15 2023-07-21 statistics ndvi_value 0 0.34161560 0.8719050
#> 6 2023-07-15 2023-07-21 statistics cloud_cover 0 18.95432692 100.0000000
#> 7 2023-07-22 2023-07-28 statistics ndvi_value 0 0.54981498 0.8600247
#> 8 2023-07-22 2023-07-28 statistics cloud_cover 0 0.04086538 1.0000000
#> stDev sampleCount noDataCount
#> 1 0.3130056 1120 704
#> 2 0.1979783 1120 704
#> 3 0.3050726 1120 704
#> 4 0.1739926 1120 704
#> 5 0.2366125 1120 704
#> 6 27.4637931 1120 704
#> 7 0.2815753 1120 704
#> 8 0.1979783 1120 704
weekly_stats_extended
#> from to output band min mean max
#> 1 2023-07-01 2023-07-07 statistics ndvi_value 0 0.59074559 0.9372641
#> 2 2023-07-01 2023-07-07 statistics cloud_cover 0 0.04086538 1.0000000
#> 3 2023-07-08 2023-07-14 statistics ndvi_value 0 0.58174862 0.9156258
#> 4 2023-07-08 2023-07-14 statistics cloud_cover 0 0.03125000 1.0000000
#> 5 2023-07-15 2023-07-21 statistics ndvi_value 0 0.34161560 0.8719050
#> 6 2023-07-15 2023-07-21 statistics cloud_cover 0 18.95432692 100.0000000
#> 7 2023-07-22 2023-07-28 statistics ndvi_value 0 0.54981498 0.8600247
#> 8 2023-07-22 2023-07-28 statistics cloud_cover 0 0.04086538 1.0000000
#> 9 2023-07-29 2023-08-04 statistics ndvi_value 0 0.57482954 0.9039569
#> 10 2023-07-29 2023-08-04 statistics cloud_cover 0 0.02403846 1.0000000
#> stDev sampleCount noDataCount
#> 1 0.3130056 1120 704
#> 2 0.1979783 1120 704
#> 3 0.3050726 1120 704
#> 4 0.1739926 1120 704
#> 5 0.2366125 1120 704
#> 6 27.4637931 1120 704
#> 7 0.2815753 1120 704
#> 8 0.1979783 1120 704
#> 9 0.2998643 1120 704
#> 10 0.1531686 1120 704
In this example we have demonstrated that a week can be specified as either 7 days or 1 week.
Retrieving statistics with percentiles
Besides the basic statistics (min, max, mean, stDev), one can also request to compute the percentiles. If the percentiles requested are 25, 50, and 75, the corresponding output is renamed ‘q1’, ‘median’, and ‘q3’.
daily_stats <- GetStatistics(aoi = aoi, time_range = c("2023-07-01", "2023-07-31"),
collection = "sentinel-2-l2a", script = script_file, mosaicking_order = "leastCC",
resolution = 100, aggregation_period = 1, percentiles = c(25, 50, 75),
client = OAuthClient)
head(daily_stats, n = 10)
#> date output band min q1 median mean
#> 1 2023-07-01 statistics ndvi_value 0 0.06187625 0.1854656 0.210216716
#> 2 2023-07-01 statistics cloud_cover 0 9.00000000 20.0000000 21.028846154
#> 3 2023-07-04 statistics ndvi_value 0 0.00000000 0.0000000 0.001150263
#> 4 2023-07-04 statistics cloud_cover 65 65.00000000 65.0000000 65.000000000
#> 5 2023-07-06 statistics ndvi_value 0 0.39318886 0.7291402 0.590745587
#> 6 2023-07-06 statistics cloud_cover 0 0.00000000 0.0000000 0.040865385
#> 7 2023-07-09 statistics ndvi_value 0 0.00000000 0.0000000 0.000207250
#> 8 2023-07-09 statistics cloud_cover 65 65.00000000 65.0000000 65.000000000
#> 9 2023-07-11 statistics ndvi_value 0 0.38260868 0.7194318 0.581748619
#> 10 2023-07-11 statistics cloud_cover 0 0.00000000 0.0000000 0.031250000
#> q3 max stDev sampleCount noDataCount
#> 1 0.3439528 0.650965929 1.700419e-01 1120 704
#> 2 32.0000000 59.000000000 1.413258e+01 1120 704
#> 3 0.0000000 0.071405768 6.540354e-03 1120 704
#> 4 65.0000000 65.000000000 0.000000e+00 1120 704
#> 5 0.8292812 0.937264144 3.130056e-01 1120 704
#> 6 0.0000000 1.000000000 1.979783e-01 1120 704
#> 7 0.0000000 0.002914758 5.075671e-04 1120 704
#> 8 65.0000000 65.000000000 0.000000e+00 1120 704
#> 9 0.8155493 0.915625751 3.050726e-01 1120 704
#> 10 0.0000000 1.000000000 1.739926e-01 1120 704
weekly_stats <- GetStatistics(aoi = aoi, time_range = c("2023-07-01", "2023-07-31"),
collection = "sentinel-2-l2a", script = script_file,mosaicking_order = "leastCC",
resolution = 100, aggregation_period = 7, percentiles = seq(10, 90, by = 10),
client = OAuthClient)
head(weekly_stats, n = 10)
#> from to output band min P.10.0 P.20.0
#> 1 2023-07-01 2023-07-07 statistics ndvi_value 0 0.00000000 0.2386117
#> 2 2023-07-01 2023-07-07 statistics cloud_cover 0 0.00000000 0.0000000
#> 3 2023-07-08 2023-07-14 statistics ndvi_value 0 0.00000000 0.2473698
#> 4 2023-07-08 2023-07-14 statistics cloud_cover 0 0.00000000 0.0000000
#> 5 2023-07-15 2023-07-21 statistics ndvi_value 0 0.03113456 0.1101370
#> 6 2023-07-15 2023-07-21 statistics cloud_cover 0 0.00000000 0.0000000
#> 7 2023-07-22 2023-07-28 statistics ndvi_value 0 0.00000000 0.2345048
#> 8 2023-07-22 2023-07-28 statistics cloud_cover 0 0.00000000 0.0000000
#> P.30.0 P.40.0 P.50.0 mean P.60.0 P.70.0 P.80.0
#> 1 0.5270270 0.6485913 0.7291402 0.59074559 0.7688466 0.8111159 0.8490484
#> 2 0.0000000 0.0000000 0.0000000 0.04086538 0.0000000 0.0000000 0.0000000
#> 3 0.5275056 0.6426682 0.7194318 0.58174862 0.7583096 0.7922882 0.8290598
#> 4 0.0000000 0.0000000 0.0000000 0.03125000 0.0000000 0.0000000 0.0000000
#> 5 0.1839369 0.2453626 0.3146195 0.34161560 0.3871180 0.4729951 0.5737004
#> 6 0.0000000 2.0000000 4.0000000 18.95432692 10.0000000 23.0000000 35.0000000
#> 7 0.5171583 0.6132419 0.6799566 0.54981498 0.7185010 0.7427998 0.7737296
#> 8 0.0000000 0.0000000 0.0000000 0.04086538 0.0000000 0.0000000 0.0000000
#> P.90.0 max stDev sampleCount noDataCount
#> 1 0.8826502 0.9372641 0.3130056 1120 704
#> 2 0.0000000 1.0000000 0.1979783 1120 704
#> 3 0.8645161 0.9156258 0.3050726 1120 704
#> 4 0.0000000 1.0000000 0.1739926 1120 704
#> 5 0.6986371 0.8719050 0.2366125 1120 704
#> 6 62.0000000 100.0000000 27.4637931 1120 704
#> 7 0.8089786 0.8600247 0.2815753 1120 704
#> 8 0.0000000 1.0000000 0.1979783 1120 704
Retrieving a series of statistics in a batch
Just as when retrieving satellite images, one can be interested in
acquiring a series of statistics for a particular zone (AOI or bounding
box) for several dates, or the statistics of different zones for the
same periods. The GetStatisticsBy*
functions
(GetStatisticsByTimerange
, GetStatisticsByAOI
,
GetStatisticsByBbox
) facilitate this task as they are
specifically crafted for being called from a lapply
-like
function, and thus potentially be executed in parallel. The following
example illustrates how to do this.
dsn <- system.file("extdata", "centralpark.geojson", package = "CDSE")
aoi <- sf::read_sf(dsn, as_tibble = FALSE)
script_file <- system.file("scripts", "NDVI_dataMask_float32.js", package = "CDSE")
seasons <- SeasonalTimerange(from = "2020-06-01", to = "2023-08-31")
lst_stats <- lapply(seasons, GetStatisticsByTimerange, aoi = aoi,
collection = "sentinel-2-l2a", script = script_file, mosaicking_order = "leastCC",
resolution = 100, aggregation_period = 7L, client = OAuthClient)
weekly_stats <- do.call(rbind, lst_stats)
weekly_stats <- weekly_stats[order(weekly_stats$from), ]
row.names(weekly_stats) <- NULL
head(weekly_stats, n = 5)
#> from to output band min mean max stDev
#> 1 2020-06-01 2020-06-07 statistics ndvi_value 0 0.5098300 0.8682218 0.2910484
#> 2 2020-06-08 2020-06-14 statistics ndvi_value 0 0.5726369 0.9431616 0.3054573
#> 3 2020-06-15 2020-06-21 statistics ndvi_value 0 0.6214251 1.0000000 0.3205804
#> 4 2020-06-22 2020-06-28 statistics ndvi_value 0 0.4591027 0.8495593 0.2735705
#> 5 2020-06-29 2020-07-05 statistics ndvi_value 0 0.5989513 0.9304222 0.3006899
#> sampleCount noDataCount
#> 1 1120 704
#> 2 1120 704
#> 3 1120 704
#> 4 1120 704
#> 5 1120 704
Copernicus Data Space Ecosystem services status
If you encounter any connection issues while using this package, please check your internet connection first. If your internet connection is working fine, you can also check the status of the Copernicus Data Space Ecosystem services by visiting this webpage. It provides a quasi real-time status of the various services provided. Once you are on the webpage, scroll down to Sentinel Hub, and pay particular attention to the Process API (used for retrieving images), Catalog API (used for catalog searches), and Statistical API (used for retrieving statistics).